Mitigating Risks in AI Project Management: Strategies for Successful AI Implementation
This article explores risk mitigation strategies for successful B2B AI projects, emphasizing the importance of AI certification for project managers.
Discover risk management strategies to mitigate risks in B2B AI projects, ensuring successful implementation and maximizing ROI.
Many businesses are enthusiastic about the promise of artificial intelligence (AI), but turning those concepts into real-world outcomes is difficult. According to Gartner, roughly only 54% of AI initiatives get from the experimental stage to full-scale deployment.
But there is good news. New AI technologies and ways of working are assisting organizations in meeting these problems. Projects that may have failed in the past now have a higher chance of succeeding and delivering the desired advantages.
In this blog, we will explore how to manage AI risks and how project managers and businesses can implement crucial measures to ensure a successful AI adoption process.
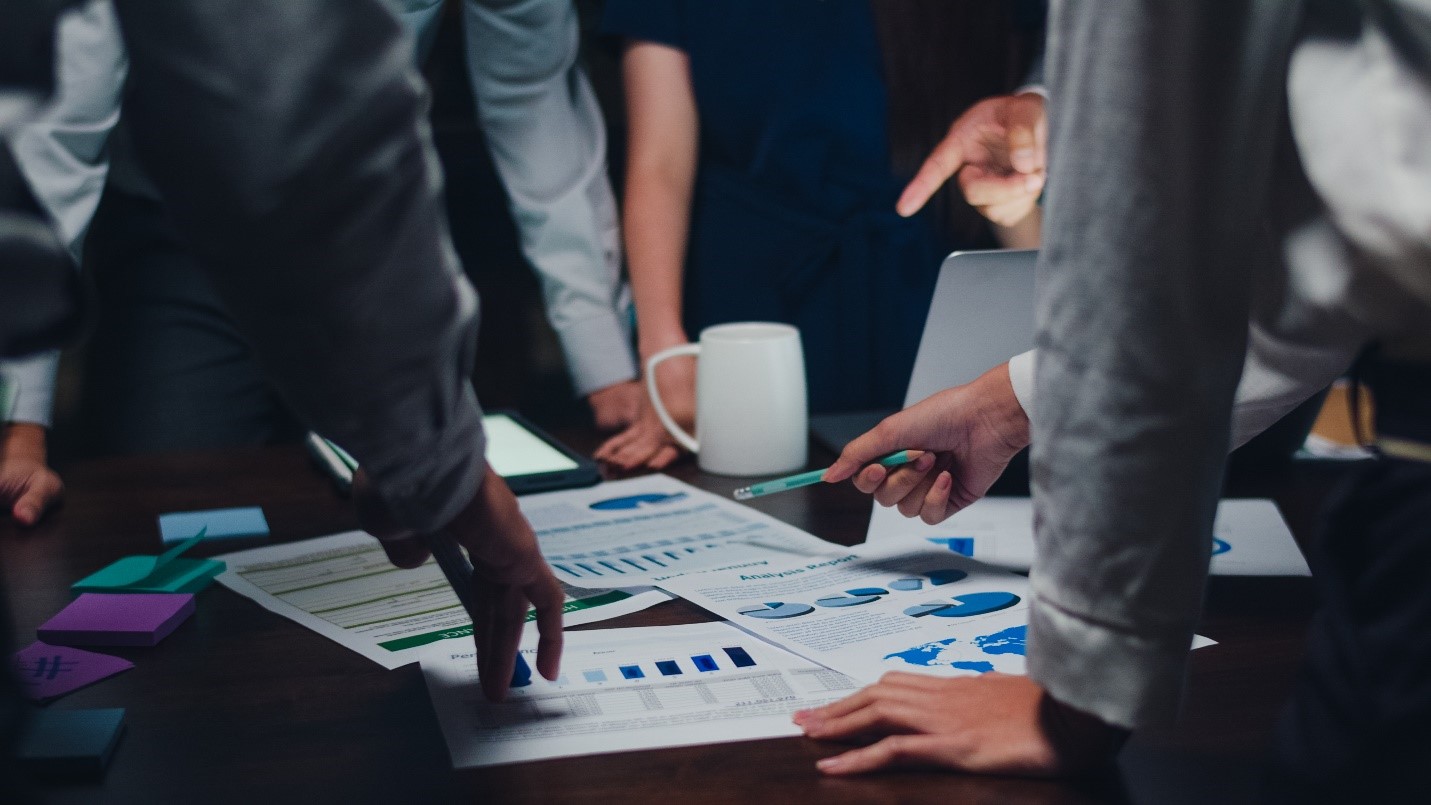
Risk in Project Management
Project risk encompasses potential challenges that could arise during project execution. Effective risk management involves identifying and addressing these challenges to enhance project success.
A risk assessment is the process of developing a list of potential risks and debating how to manage them. All members of the project team must conduct risk analysis, which includes holding meetings to gather information about potential risks.
Risks can have an impact on people, processes, technology, and resources, but they differ from issues, which are foreseeable events. Risks, however, are unanticipated events like flu season or product backorders.
Steps to Identify the Risks in Project Management
When assessing the risk in project management, we need to ask the following questions:
1. What could happen to your project?
2. When is it likely to occur?
3. What are the possibilities of this happening?
4. What is the expected result?
5. What occurrences could initiate the risk event?
We have identified five crucial factors to consider when calculating project risk. Now, let us address some prevalent misconceptions about AI project risk management.
Misconceptions regarding AI Project Risk Management
- AI project risk management is an unnecessary expense.
- Reality: Effective risk management involves identifying potential dangers, assessing their impact, and adopting mitigation strategies. It helps prevent costly project delays and failures while also creating trust among stakeholders.
- AI project risk management is limited to large-scale initiatives.
- Reality: Effective risk management is crucial for any project, including small AI programs. It aids in reducing risks such as data breaches, algorithmic biases, and insufficient testing.
- AI project risk management removes all hazards.
- Reality: Risk management focuses on discovering, evaluating, and reducing hazards rather than removing them entirely. It reduces the possibility of risks occurring and ensures proactive risk management throughout the project’s lifecycle.
- AI project risk management is a one-time operation.
- Reality: AI project risk management is a continuous process that should be integrated throughout the project’s lifecycle to detect new risks, ensure adaptability, and monitor for timely changes.
- AI project risk management is exclusively the duty of the project manager.
- Reality: Effective AI project risk management requires a collaborative effort from all stakeholders, including team members, AI experts, data scientists, and business analysts, each offering their unique knowledge and insights.
Moving beyond these myths, let’s look at how AI might be a useful tool for identifying and prioritizing risks in your AI initiatives.
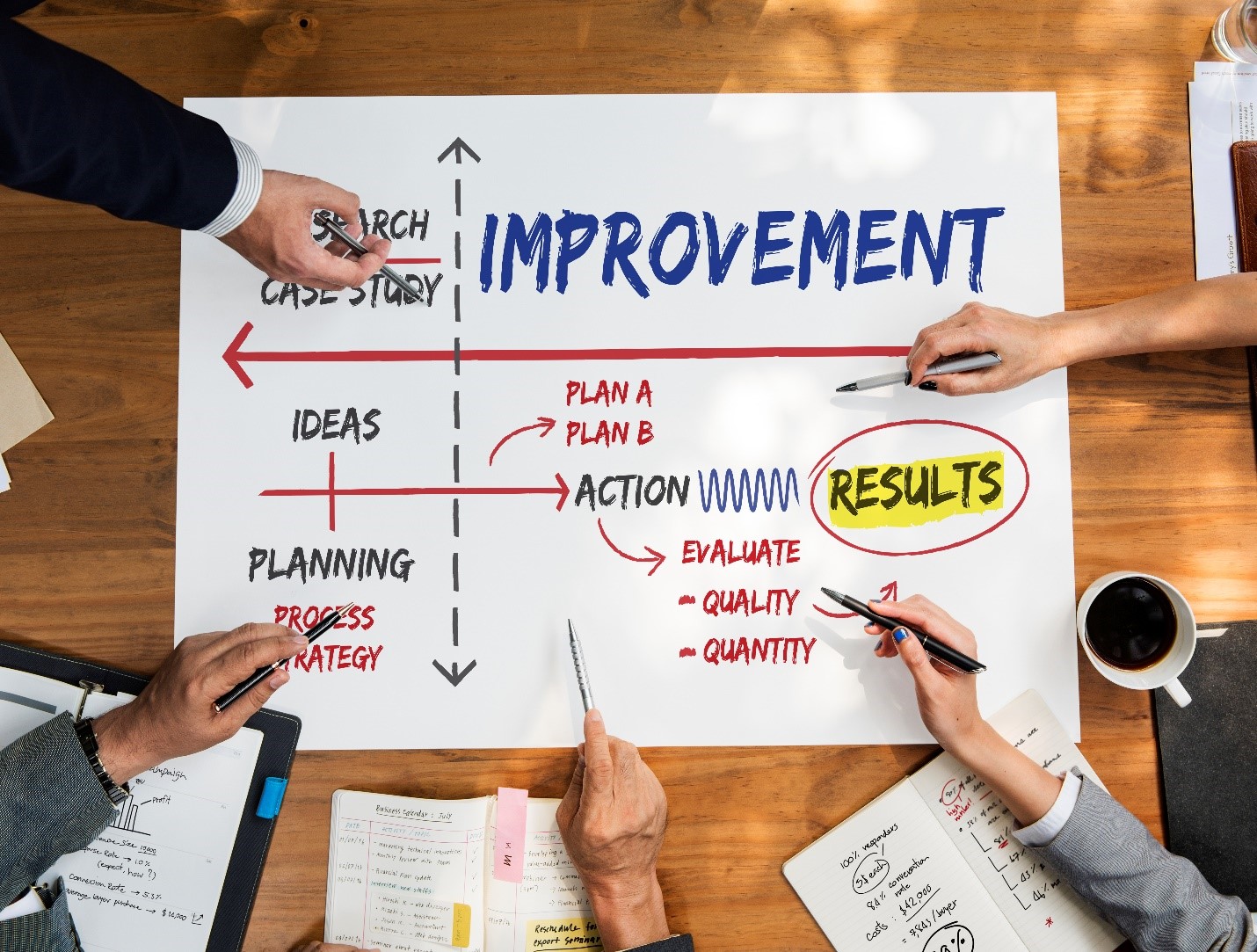
AI Risk Detection and Prioritization
AI adds substantial benefits to enterprises, but it also introduces several hazards and ethical issues, which authorities are scrutinizing and potentially penalizing. To effectively manage AI risks, firms should place legal and risk-management teams at the center of the AI development process, including data collection and governance.
An intelligent risk-prioritization strategy based on legal guidance and technical best practices is required. This requires building a library of unique AI risks and following a clear methodology to analyze and prioritize such risks for mitigation.
Policymakers are tackling dangers connected with AI initiatives, including ethical concerns, algorithm bias, and security flaws.
One such methodology is McKinsey & Company’s, which focuses on ensuring that AI models comply with societal standards and regulatory requirements. This methodology includes a framework for AI models that is focused on results while also addressing potential AI-related issues such as privacy and fairness. A responsible AI program reduces risks by integrating policies, oversight, and tools to ensure that AI aligns with company values and ethical behavior.
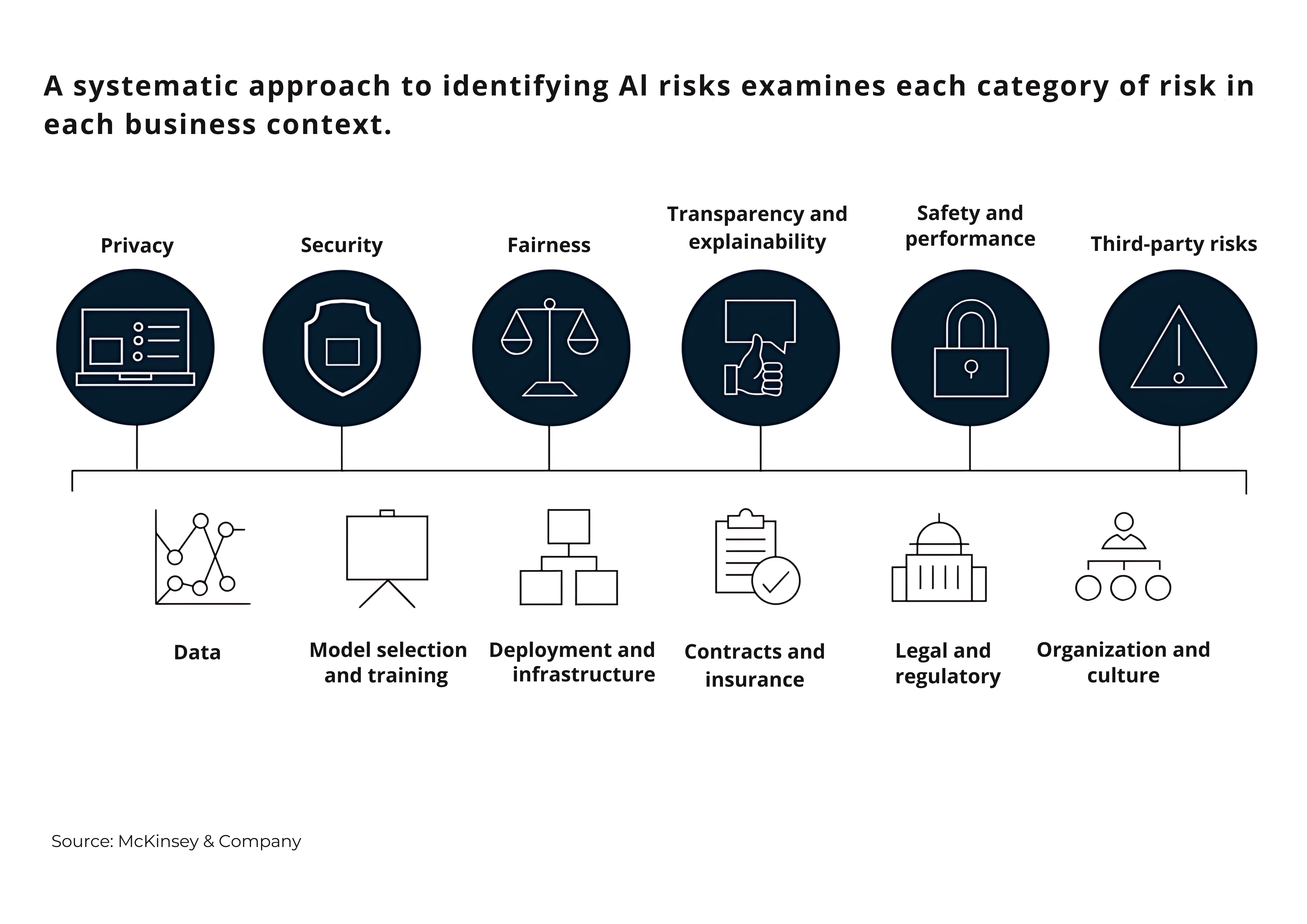
Building on the necessity of risk management, let’s look at some important strategies for achieving effective AI deployment.
Strategies for Successful AI Implementation
To successfully implement AI, we must manage AI risk, define the context and objectives, identify AI risks, monitor and audit them regularly, evaluate each potential risk, and implement mitigation solutions. These techniques encompass preventative, detection, and correction approaches. Organizations can use tools like risk matrices and decision trees to prioritize risks and make informed decisions.
Risk management requires expert guidance, historical data, risk responses, risk owners, and resources. Monitoring ensures plan implementation and addresses all risks, including positive ones. Let’s get started on developing a comprehensive risk management strategy for your AI project.
Create a Risk Management Plan
A risk management strategy is necessary for project managers to document, plan for, and limit any risks to the project’s execution and completion. It should include a risk breakdown structure that divides hazards into known, unknown, and unknowable categories. Each risk should be fully defined, including its consequences and likelihood of occurrence. Once the plan is created, assign risk ownership and create proactive risk responses.
To remain competitive, project managers must adapt to artificial intelligence. They should invest in continual learning and skill development to stay current on AI breakthroughs and capabilities. They should also view AI as a collaborative partner, providing valuable assistance with decision-making, risk management, and resource allocation. Understanding AI’s capabilities and limitations enables project managers to exploit their strengths for project success.
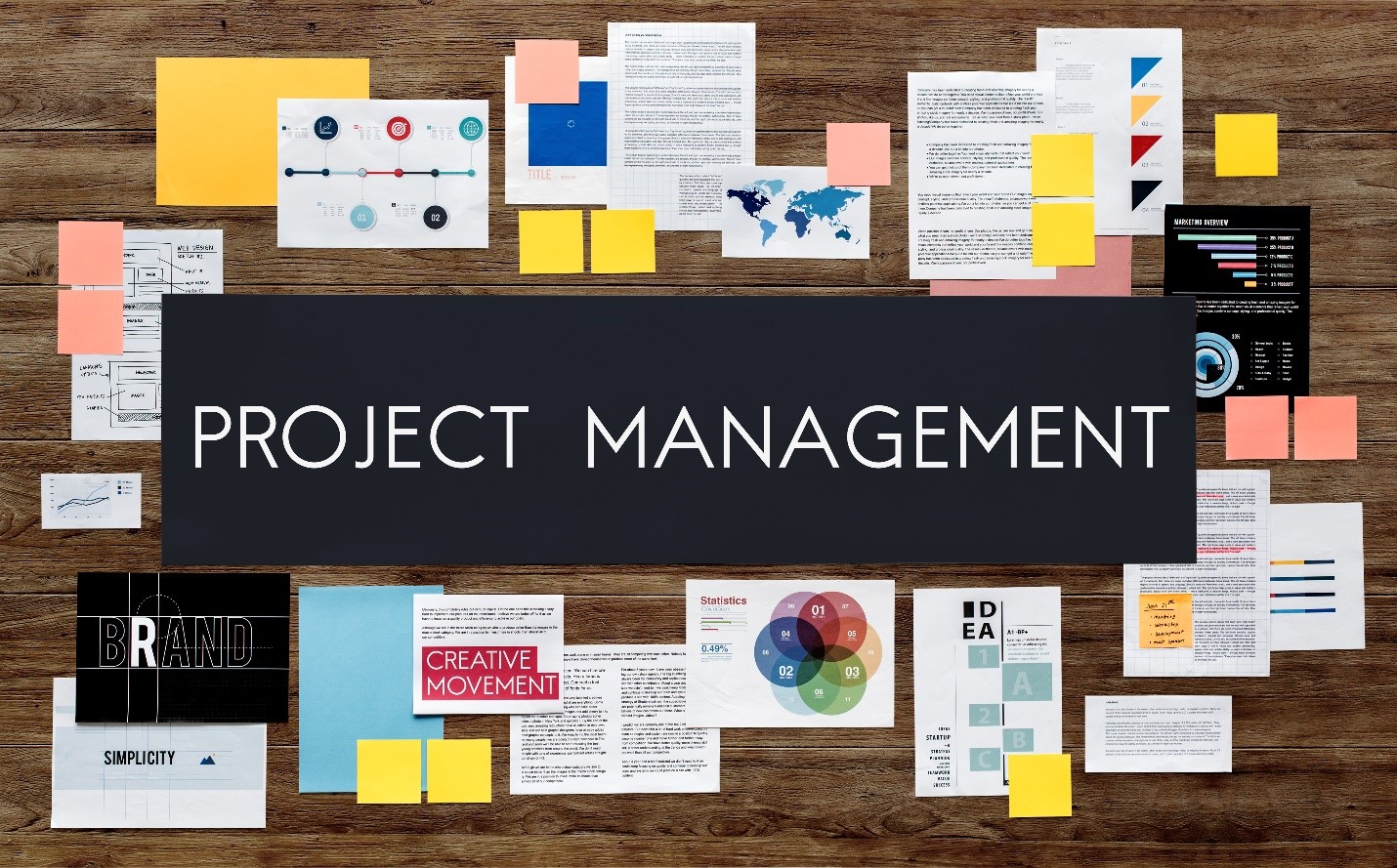
Takeaways
1. The Importance of Risk Management: Poor risk management results in AI project failures. Proactive risk identification and mitigation are critical.
2. Key Steps to Effective Risk Management: Define project objectives, identify possible risks, prioritize them, devise mitigation techniques, and monitor and adjust the risk management strategy.
3. AI-Specific Risk Considerations: Ethical issues and algorithmic prejudice are distinct risks in AI research. Legal and regulatory compliance must be considered.
4. Project Manager’s Role in the AI Era: Adapt your abilities to keep up with AI breakthroughs and consider AI as a collaborative tool.
5. Additional Advice: Use risk management tools, invest in training, and pursue AI certifications.
Conclusion
Effective risk mitigation in AI project management involves utilizing AI technologies to predict possible hazards in real-time, optimize resources, and meet project objectives. By using AI, businesses may drastically reduce failure rates, improve project results, and increase overall efficiency. By implementing these risk mitigation techniques, businesses can drastically limit the negative implications of AI initiatives while ensuring their success. Furthermore, enrolling in the AI CERTs™’ AI+ Project Manager™ certification can help project managers comprehend and apply AI concepts to real-world risk mitigation challenges.
You may also learn more in the blog ‘Building High-Performing AI Teams: Bridging the Business-Tech Divide.‘